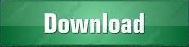
In addition, research challenges are discussed, with focus on developments on different big data framework, such as Hadoop, Spark and Flink and the encouragement in devoting substantial research efforts in some families of data preprocessing methods and applications on new big data learning paradigms. The connection between big data and data preprocessing throughout all families of methods and big data technologies are also examined, including a review of the state-of-the-art. The definition, characteristics, and categorization of data preprocessing approaches in big data are introduced. The presence of data preprocessing methods for data mining in big data is reviewed in this paper. Addressing big data is a challenging and time-demanding task that requires a large computational infrastructure to ensure successful data processing and analysis. Big Data can be defined as high volume, velocity and variety of data that require a new high-performance processing. add( i, var * np.The massive growth in the scale of data has been observed in recent years being a key factor of the Big Data scenario. uniform( 0, 1) # Generate the variance multiplier, float type synthetic. subtract( data], i) # Difference between two closely related samples var = rd. randrange( 0, k) # Randomly select one of the nearest neighbor, integer type diff = np. remove( nn_index)Ĭount = count + 1 # Synthesize data for data sample "i": while mul != 0: append( nn_index) # Record the indices corresponding to nearest neighbor nn_index. # Keep the first few nearest neighbors based on k: count = 0 while count < k: # this if-condition removes bugs when user purposely define higher k than the available data samples: if k > len( data): Mul = int( n / 100) # integer multiple of the percentage chosen nn_array = list() # array to store the indices of nearest neighbors for sample "i" nn_val = list() # temporary list to store euclidean distance value for future comparison nn_index = list() # temporary store indices for all neighbors of sample "i" # Computing euclidean distance between sample "i" and the rest of the data samples: for ind, dt in enumerate( data):Ĭontinue # do not compute nearest neighbors for same data else: # Algorithm for SMOTE: synthetic = list()
Smote data creator manual#
The written code below separates the data variables and the class label, hence no manual separation needed Number of nearest neighbor, k : Default k is 5.For nx100%, each data sample is synthesized n times, hence size of synthetic data is n times bigger than input dataset. If the size is smaller than 100%, for instance 50%, this means only 50% of the input dataset is used to synthesize data once. By default, this argument value os 100%, which means each data sample is synthesized once and the number of synthesized data is equal to length(input dataset). Amount of synthesis (size) : This is in percentage format, ie : 20, 50, 100, 200, 400, 600.Need not separate data variables from its class label. , ], attr is the data attribute/variable. Rundown of Code Input Arguments for the Algorithm al 2002, hence the name, Synthetic Minority Oversampling Technique (SMOTE).

Oversampling the minority class is like data augmentation, which in this case is done by synthesizing data using given data input, as proposed by Chawla et. To solve this issue, one could undersample the majority class or oversample the minority class. For example, when I was using CART or decision tree to classify breast cancer cells as benign or malignant with a class-imbalance dataset, I notice the classifier made a one-sided prediction on my validation data, eventhough the data contains two different classes.
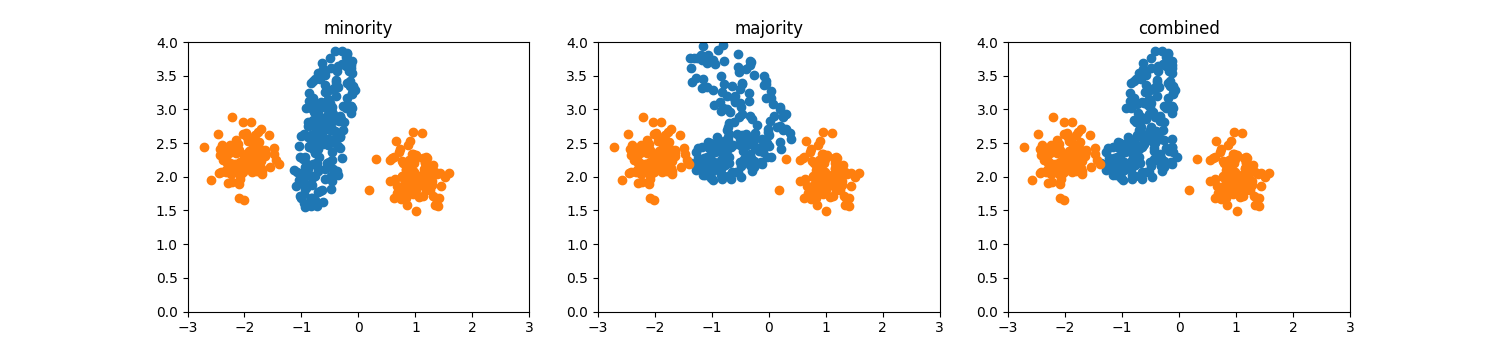
This creates a bias whereby the classifier favors the majority class. For example, a binary class dataset could contain 100,000 data samples but only 1,000 of them represents a particular data class whereas the rest are the opposite class. When dealing with large datasets, it is common to stumbled on uneven proportion of data classes. A minority oversampling method for imbalance data set Brief Context on SMOTE
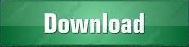